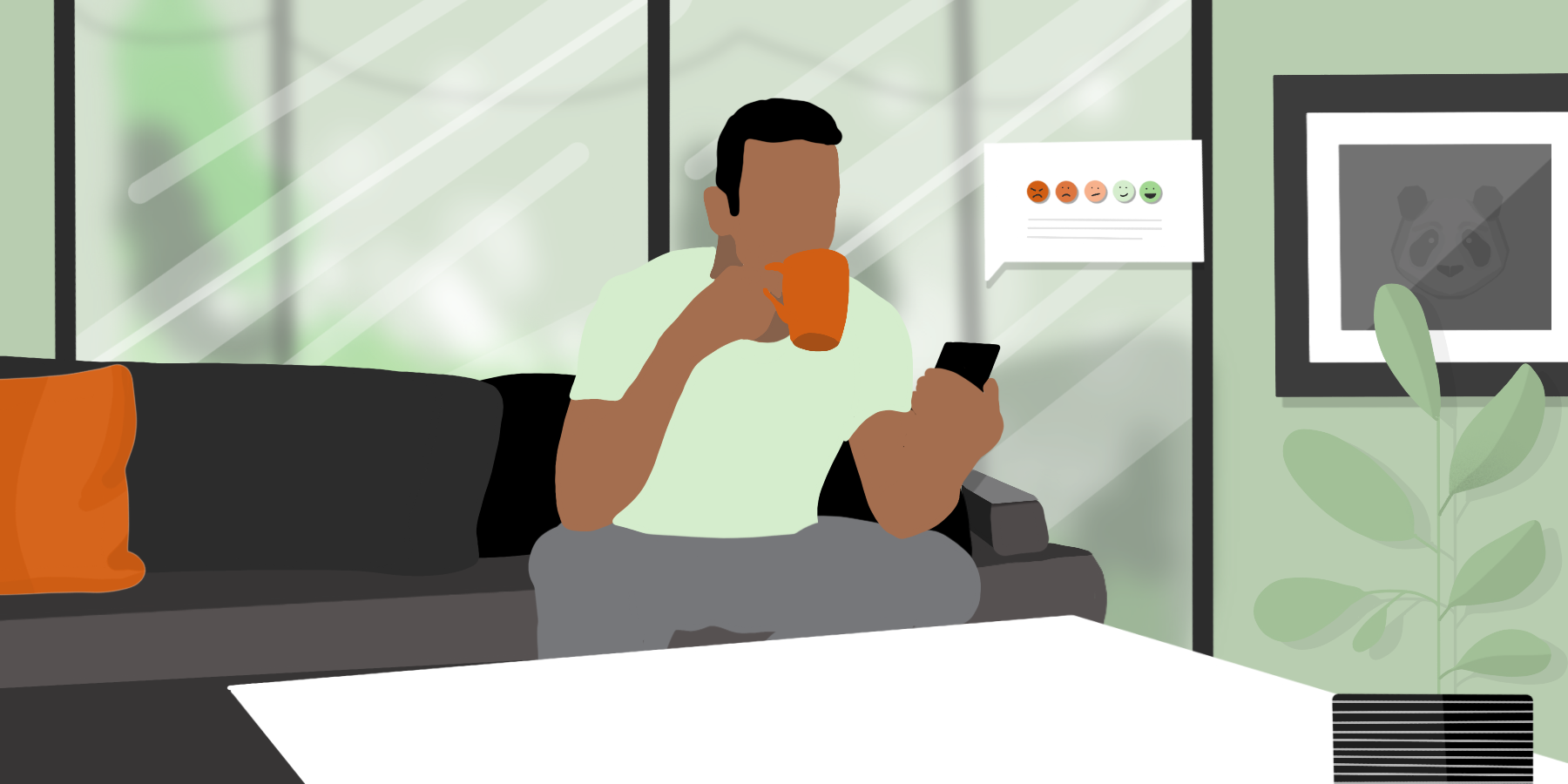
How to Get Greater Intelligence From Your Marketing Analytics
Any entry-level marketer can tell you that it’s important to make decisions based on data. In practice, even the best marketing teams often find themselves making decisions based on hypotheses.
Leveraging data to its fullest potential allows your team to increase personalization, improve the customer journey, and make smarter business decisions that lead to a higher ROI.
In order to get the most out of business intelligence and marketing analytics, your team needs a holistic approach to data collection and analysis. Simply put, once you have your data, how do you turn it into an actionable, executable marketing plan?
In this article, we’ll show you exactly how to gather meaningful data and effectively analyze it.
Table of contents
Business intelligence strategy (gathering data effectively)
There are two kinds of business intelligence (BI) data that will inform your marketing strategy. While we think it’s safe to assume you know the difference between quantitative and qualitative data, let’s take a look at some of the ways they manifest specifically within marketing tools.
Quantitative data, or that which can be measured or counted, is often the information that lives within your marketing technology (martech) stack. Think of the data you find when you pull up Google Analytics or the click and open rates you see within your email service provider.
Quantitative data your team may be interested in includes:
- Web analytics
- Campaign analytics
- Content performance
- Audience reach
- Conversion rates
On the other hand, qualitative data is less likely to live within a system because it tends to come from human emotion or sentiment. How do people feel or think about your brand, campaign, content, user journeys, digital experience?
Examples of qualitative marketing data include:
- Social proof
- Audience engagement (while, say, the number of shares is quantitative, are people retweeting your post on social media platforms because they love it, or sharing it with negative commentary to express a grievance?)
- Brand loyalty
- Customer interviews
- Focus group feedback
When you look at all of the quantitative and qualitative data available to marketers, it’s obvious that marketing analytics is a huge undertaking.
It’s important to remember that you don’t need to collect every type of data for every project your team works on. Sometimes the numbers speak for themselves, as might your customers. There’s no need to gather data for data’s sake.
What is meaningful data?
Here’s an obvious statement: collect only the data you need to help you make meaningful business and marketing decisions.
But what’s less obvious is how to decipher what data counts as meaningful and then how to collect it. If you don’t know why you’re measuring something or what you want to learn, the data sources you have in place may not aid decision-making.
Meaningful data helps you and your team answer questions and test hypotheses. It also informs strategy and business decisions. For example:
- A content marketer might use analytics to understand which topics to blog about, as well as which distribution and marketing channels will be most effective for reaching their target audience.
- An email marketer likely analyzes send time and frequency based on meaningful data like click and open rates.
- A social media marketer will want to lean on both quantitative and qualitative data (as explained in the audience engagement example above).
When customer data helps to explain the reason behind your audience’s behavior (and the actions they take), you can meaningfully understand what marketing campaigns are (or aren’t) working. Then, optimize to improve your customer experience, conversion rate, and ultimately ROI.
When and how to collect data
Before you collect data, ask yourself:
- What do I want to find out?
- What KPIs do I want to track and inform?
- Who will use our data in the end-stage?
- How can we ensure data quality and accuracy?
- What is our plan for analyzing our findings and implementing our learnings?
Once you’re sure there’s intent behind the data you plan to collect, it’s important to think through when and how you’ll collect it.
Since the foundation of customer loyalty is trust, you need to be careful not to over-ask your audience for data or to ask at the wrong time. Even your company’s strongest advocate is not interested in frequent surveys and weekly chats with your customer success team.
As a consumer, it can also feel awkward to hear from a company out of the blue, so it’s best to ask for what you need after the customer triggers an action.
For example, you may send out a quick customer satisfaction survey (CSAT) or net promoter score (NPS) questionnaire right after a purchase has been made. This way, you’re striking while the iron is hot, so to speak, and leveraging a response while they feel delighted and satisfied with their experience.
Similarly, if a customer has just left you a negative review, there’s a fine line between ignoring it and jumping on the phone while the customer is likely still heated. You probably won’t have a positive conversation or glean much of a takeaway immediately after a negative review is left.
But to rebuild trust, it is important to reach out within a few days and lead with empathy. This way, you acknowledge their negative experience and show that you care about making it right.
If you proceed in this fashion, you can learn incredibly useful information that will help you identify what went wrong and fix any digital marketing support gaps to avoid recurring issues.
Besides asking customers to give you their feedback outright, you can also test your marketing initiatives behind the scenes (such as A/B testing landing pages or CTAs). There are advanced tools at your disposal to help you out, and we’ll dive into how to leverage them in the final section of this article.
How to use your data intelligently
Depending on your experience, it may or may not surprise you to hear that only 54% of marketing decisions are made based on analytics.
While we may intuitively understand the importance of data, not every individual marketer or marketing team has the skills to effectively analyze it. Data isn’t just about collecting information, you need to then use it to improve your business and marketing.
Once you’re clear on what you’re measuring and why there are a few ways to go about using your data intelligently.
Build reports and action your results
Create reports that make it easy to segment data by the metrics that matter based on your goals, benchmarks, and KPIs. How you structure it will depend entirely on the answers to the questions above.
Once you’ve built a report, make sure it’s accessible to the right team members (such as with custom marketing dashboards tailored with the data that matters to the stakeholders and team members at hand). Write an abstract so team members understand the visualization they’re looking at, where to find it, and when and why they should plan to access it.
Once you know what you’re looking for and how you want to segment it, you then need to understand how to action those results meaningfully.
For example, if a report says X and your goal was Y, it’s critical to look at the data for answers about what went wrong, how your initiative deviated from the plan, or why it didn’t resonate as expected.
Depending on the type of data you’re collecting and whether it’s one-off or ongoing, consider including data analysis in recurring team meetings, postmortems, etc. These actionable insights can (and should, in many cases) be shared with stakeholders and your internal team.
Recruit the right talent
Data analytics is not going to be every marketer’s forte. It’s critical to ensure you have the right talent in place to maximize your data analytics tools.
While every marketer should have a baseline understanding of how data drives marketing decisions, you need people who do more than basic data processing. Tools are important, but understanding how to make sense of data requires an important human touch.
Given that this analysis will go on to inform some of your most critical marketing strategy and business decisions, it’s important to entrust this job into highly capable hands.
Once you have the right tool(s) in place, you’ll want the process of updating and sharing it to be as automated as possible. From there, the right people (that fully understand your digital customer experience, workflows, processes, etc.) can quickly decipher what the data means and how to implement any findings.
You can see how, even with an excellent digital experience platform providing accurate, up-to-date data, if no one is looking at it or interpreting it through the right lens, there’s room for improvement.
How to leverage emerging business intelligence tools and trends
If any (or all!) of this information seems overwhelming, not to worry. There are plenty of emerging BI tools and trends out there to help you and your team excel at business intelligence analysis.
Meanwhile, here are some of the ways automation tools can help your team excel at business intelligence collection and analysis:
Machine learning
Machine learning helps you align with your customers more effectively. It can inform which actions to take and when to get the right message in front of them at the right time.
This approach helps remove the guesswork from marketing decisions so you can focus on data-forward decisions and strategies. Importantly, machine learning-based algorithms remove human bias from data interpretation.
While it’s important that your people understand data and its implications, it’s obviously not scalable in every situation for every data set, and there are cases where removing bias may be more important than others.
For example, if your team is creating content around a culturally sensitive topic or movement, it’s probably not enough to just look at the numbers. Humans can understand nuance much better than machines, so you wouldn’t want to glean customer reaction from numbers alone.
You can also leverage machine learning as a form of predictive analytics. By analyzing historical and current data, AI and machine learning can help you make important predictions and forecasts about future outcomes.
For example, healthcare organizations can use big data to provide better care and services. This may look like identifying patients that are at a higher risk for developing a chronic condition based on their history and publicly available information and studies.
Doctors and clinicians can then apply this knowledge to patient care by creating a preventative treatment plan or advising certain wellness activities to stave risk.
Phoenix Children’s Hospital has been using predictive analytics to reduce the incidence and severity of acute kidney injury (AKI). They’ve set up a dashboard that displays color-coded risk scores every six hours to providers overseeing at-risk patients. This allows them to more easily monitor their kidney function and adjust medication as needed.
Leveraging data in this way has the potential to save lives. Getting the word out about specialized care, therefore, is key. Understanding your target audience (in this case, children at risk for kidney failure), is of the utmost importance so that you can make them aware of your top-notch care, get them in the door, and work your magic.
Segmentation
Segmenting your audience can help automate your data by differentiating between audience types.
For example, segment your audience into buyer vs. non-buyer tracks. Look at the data within the two funnels to curate a hypothesis as to why non-buyers aren’t buying (the barrier could be budget, timing, non-personalization, etc.). From there you can test new messaging based on your hypothesis.
We did just this for Viceroy Hotels and Resorts. By using an A/B test to understand customer behavior when it comes to booking a room, we pitted five calls to action (CTA) buttons against each other. There was a clear winner, and this simple segmentation test increased room reservations by $30,000 per month.
Segmentation can also be randomized; for example, showing ads to one group and not the other and comparing behavior between the two.
Customer lifetime value
Understanding your customer lifetime value—and having an automated system for tracking it—is critical for pretty much all business and marketing decisions.
In order to forecast successfully, you’ll want to know things like:
- Customer acquisition cost (CAC)
- Customer lifecycle (are your ideal customer profiles one-time buyers or is your business based on retention and recurring revenue?)
- Opportunity for upselling or add-ons
Knowing how much your customers are paying you—and when and for how long you can expect that revenue—informs your bottom line.
Having this data automated makes it easy to plug in different numbers (say, if you’re planning a discount or price increase) so you can easily see how changing one number will affect the others.
Customer attribution
It’s critical for marketers to always have a pulse on where customers are coming from. Automating your customer attribution systems can help you optimize return on initiatives like ad spend while using less budget.
By testing different copy on various ads and landing pages, we helped LoJack Corporation identify where customers were coming from. Getting clear on customer attribution increased their conversions by 59%.
How to run meaningful experiments
There’s a lot at stake when it comes to understanding who your customer is, where they came from, and what they are (or may be) worth. Artificial intelligence (AI) helps us do this in real-time—and will only continue to help marketers understand and predict customer behavior.
While AI offers enormous value to marketing professionals, remember what we said earlier about needing the right tools and the right people?
As with all of the most successful marketing departments, the human element is critical to being able to engage with, understand, and meet your customer where they are, at the right time.
Leveraging these tools and trends is important, but it’s still important to make sure you’re asking the right questions and running the right experiments.
At Smart Panda Labs, we refer to this idea of experimentation as Build, Measure, Learn, or BML. There is no one size fits all approach among the teams we work with and each step may vary in length depending on the size of the data set, traffic, etc.
BML is circular, so once you learn something, you go back to the start and build and optimize to drive continuous improvement.
Naturally, the data sample size will also vary from client to client. For instance, when measuring experiments for clients with lower website traffic levels:
- The test will either have to run for longer (in order to gather statistically significant data), or;
- The change will need to be designed in such a way that the impact (positive or negative) is a bigger variance from the control.
This sometimes means for clients with smaller amounts of traffic or data, the BML cycle may take longer because it will either:
- Take time to build a more complicated change to the page, or;
- Take longer to measure smaller changes in order to collect a large enough sample size
The key is that it’s a repetitive process, and knowing which stage you’re in keeps everyone apprised of whether the focus is on building, measuring, or implementing learnings.
Key takeaways
While just about every marketing team has access to data, not all analytics are equally helpful, important, or easily understood.
When considering the impact data can have on your business and strategy, first and foremost, it’s about asking the right questions and having the right team in place.
Once you collect data, it’s crucial that all relevant team members and stakeholders have access to it as well as an understanding of why it’s important. Understanding this piece is the necessary foundation for any strategies that come from your learnings.
There are plenty of tools and systems to help automate, gather, and analyze your data so you can turn it into business intelligence.
SUBSCRIBE TO OUR BLOG DIGEST!
Submit this form to receive a monthly, curated digest of our blogs, filled with helpful thought leadership, innovative trends and industry insights, all delivered straight to your inbox.